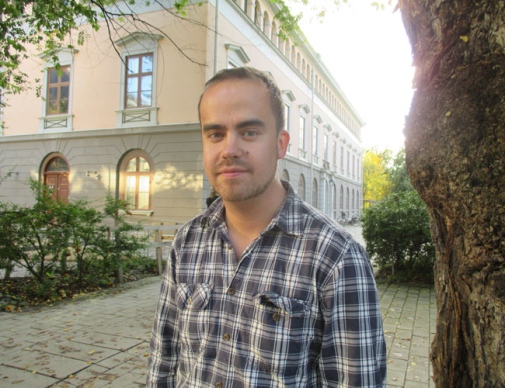
Imagine that you are going to buy certain shares tomorrow. You ask a statistician if they can analyse how the share price will behave in the future. Previously, you will have heard the answer, “Yes, I can do that – but it will take many hours to calculate this on the computer because there is so much data”. The problem is, you needed this analysis much sooner.
This is how Matias Quiroz – recently awarded his PhD – describes the research situation before he wrote his doctoral thesis, “Bayesian Inference in Large Data Problems”. One part of the thesis that can be seen as an important contribution to Big Data research deals with how large amounts of data can be analysed faster.
The algorithm is a workhorse
One common tool for analysing data within Bayesian statistics is known as the “MCMC algorithm” – MCMC being an abbreviation for Markov Chain Monte Carlo. Matias Quiroz describes this as a workhorse.
“It's standard, it's what people work with. The problem is that this method is very calculation-intensive, so many are asking if it is sustainable now and in the future,” he says.
Current MCMC research within large data amounts can be crudely divided into two areas.
“One way to use MCMC for large amounts of data is known as 'divide and conquer' This is when you divide up the data material and let many computers work with it. Once they have finished working, you gather together the information to reach conclusions,” says Matias Quiroz.
The other way, the one he is working with, is to speed up the MCMC algorithm by only using small random samples of data.
Big Data makes the workhorse sweaty
In the first of his thesis articles, Matias Quiroz – who also works with the Research Division at the Riksbank – wanted to create an interesting statistical model to apply to bankruptcy data. It tries to model the probability of companies going bankrupt, depending on what their accounts show. As MCMC is so computation-heavy, he was only able to analyse a small portion of the data material in the first article.
To continue the research, he realised that he needed to speed up the algorithms he was working with. This made him switch the focus of his thesis to focus on speeding up the algorithms instead. To put it simply, it is about how to reach a conclusion that applies to all the enormous data material – just by looking at a random sample.
“We take a subset of the observations, but we don't lose any precision in our estimates observed in the entire data material,” explains Matias, who emphasises that he has done much of the work together with others.
Here he saw the potential to connect two separate areas within statistics: MCMC methods from Bayesian inference and classic selection methodology.
Good predictions despite small random samples
To be able to choose a small random sample but maintain a lot of information, Matias Quiroz needed to choose the data observations that were the most informative.
“This is where the selection methodology comes in, because you cannot choose the data points with the same probability of being selected for everything. Instead, we develop a measure to be able to see which of the observations are the influential ones. Then, we sample the influential observations with a higher probability”.
It became clear that the methods he was using were approximations (similar but not equal). This in turn meant that he needed to make a theoretical framework to ensure that the approximations were not totally off.
“So a big part of the thesis has been devoted to creating a theoretical framework for doing MCMC method based random samples of data. This is exactly the idea of the thesis."
Facts: Bayesian statistics for dummies
In Bayesian statistics you use a model where all of its parameters are seen as random.
You could say that this is the opposite to classic statistics (frequency statistics) – where data is seen as random, but the parameters are known. In other words, the parameters aren't random, they are constant.
The Bayesian framework just swaps this reasoning around. You treat what has been seen (the data) as “known” and what hasn't been seen (the parameters) as “unknown”. They use data to update their knowledge about the parameters.
Examples of parameters include the mean value, the median and so on. To put it simply, Bayesians treat what they don't know about as random.