The external reviewer (Opponent) will be: Professor Domenico Giannone, CEPR (Centre for Economic Policy Research) och Amazon, Seattle, Washington, USA.
Oskar's Main Supervisor is Pär Stockhammar and Supervisor is Frank Miller.
To visit the dissertation, please contact Håkan Slättman for an updated zoom-link.
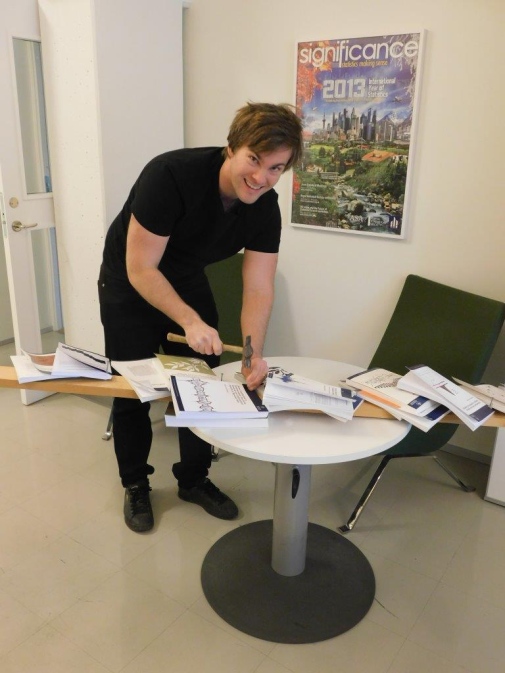
Abstract
Time-dependent volatility clustering (or heteroscedasticity) in macroeconomic and financial time series has been analyzed for more than half a century. The inefficiencies it causes in various inference procedures are well known and understood. Despite this, heteroscedasticity is surprisingly often neglected in practical work. The correct way is to model the variance jointly with the other properties of the time series by using some of the many methods available in the literature. In the first two papers of this thesis, we explore a third option, that is rarely used in the literature, in which we first remove the heteroscedasticity and only then fit a simpler model to the homogenized data.
In the first paper, we introduce a filter that removes heteroscedasticity from simulated data without affecting other time series properties. We show that filtering the data leads to efficiency gains when estimating parameters in ARMA models, and in some cases to higher forecast precision for US GDP growth.
The work of the first paper is extended to the case of multivariate time series in Paper II. In this paper, the stochastic volatility model is used for tracking the latent evolution of the time series variances. Also in this scenario variance stabilization offers efficiency gains when estimating model parameters.
During the last decade, there has been an increasing interest in using large-scale VARs together with Bayesian shrinkage methods. The rich parameterization together with the need for simulations methods results in a computational bottleneck that either force concessions regarding the flexibility of the model or the size of the data set. In the last two papers, we address these issues with methods from the machine learning literature.
In Paper III, we develop a new Bayesian optimization strategy for finding optimal hyperparameters for econometric models via maximization of the marginal likelihood. We illustrate that the algorithm finds optimal values fast compared to conventional methods.